A 10 step guide to Machine Learning success
Machine learning (ML) has the power to take an organization's digital transformation to dizzying new heights. While this is a well known fact amongst business leaders in the enterprise, full scale ML implementation is often perceived as unattainable. This, however, could not be further from the truth. For those that are open to new ways of thinking, the endless possibilities created by even minor ML deployments - such as decreased costs and helping teams to work more efficiently - are up for grabs. And many business leaders are seizing the opportunity to integrate it into their current IT infrastructure. In fact, according to Forbes research, the global ML market was valued at $1.58B in 2017 and is expected to reach $20.83B in 2024.
Santiago Giraldo, Director of Product Marketing at Cloudera.
To reap the benefits of ML, businesses must embrace a fresh approach to their data journey. At the same time, enterprises have to acknowledge one of the most common stumbling blocks they will face when it comes to ML is that implementation is not always straightforward. Often, issues arise when teams try to bridge the gap from simply trying ML to mastering full-scale ML production. While properly adopting ML requires a long-term commitment across your organization - and can frankly feel quite daunting - every organization can set themselves up for success with these ten easy-to-follow steps.
Adopting a holistic approach
Business leaders have to have the right mindset and take a holistic approach when adopting ML models. For ML to become the instigator of change, it has to be baked in from the beginning and considered a fundamental part of data strategy. When this occurs, ML is run in conjunction with existing IT environments, applications, processes and workflows, and in turn organizations can drive better business results. It is for this reason that ML must be considered as a continuous learning and developing platform from the get-go. This will ensure it is working to the best of its ability early on.
Breaking down barriers with flexibility
Organizations that have already dipped their toes in the ML waters will have observed that there is a barrier between ML experimentation and large-scale adoption. Barriers often occur when a business lacks the skills and knowledge needed to integrate ML production, maintenance and development into the existing workflows, processes, culture and architecture. It is also the reason why when tackling ML head-on, businesses need to be flexible in their approach to not only managing data but how their teams are structured. Data engineers and scientists need to work closely with leaders, to guide them on the best path to manage data, and use the insights gathered to guide the business forward.
Creating a multi-disciplined team
Crucial to the success of ML implementation is understanding that people are just as important as the technology itself. Building a team that can support ML models in their day-to-day functions, collaboration and freedom from organizational restriction are key. While leaders will want to see the ROI from integrating ML from the beginning, on the flip side, data scientists will want a platform and tools that enable them practical access to data, libraries and resources without feeling confined by access barriers and red tape. Unifying teams from a variety of disciplines means that ML models can ultimately answer various organizational needs and better power business decisions.
Get experimenting and embrace failure
From solving business problems to automating processes, there is a range of benefits that result from ML adoption. However, while it’s often these benefits that people shout about first, at its core, ML is about science - something people often forget. First and foremost, accurate science involves observation and experimentation, as well as readiness to embrace failures and successes. When it comes to ML, fortunately, even the failures can be perceived as wins. As soon as an enterprise knows a specific business problem cannot be solved with ML, that knowledge frees up efforts to be channeled into other areas. Every experiment is an opportunity to learn, and the lessons should form the foundations of future data strategies.
Rapid iteration
A common mistake many businesses make when starting their journey into ML is rushing to create a model that is completely flawless from the beginning. To avoid this, businesses need to recognize that finding your feet with ML is a process – one that requires an openness to letting teams experiment quickly, frequently fail, continuously learn, and test new things. In carrying out this iteration, organizations can learn when ML models are performing optimally for the business, powered by the right data and insights to propel the enterprise forward.
Optimizing the data lifecycle with the right technology
An additional aspect of developing ML models is having the right technology to optimize the data lifecycle. It is vital that data science and data engineering teams have the ability to control and work across the entire journey of an ML model.
This lifecycle can be split into two stages:
- The building of ML models and holistic ML development
- Getting to production scaling and ongoing operations
With the right tools and platform in place, teams will be empowered to operate seamlessly across both of these phases. This will ensure that ML models are put into production at the right time, built accurately and scaled in alignment with the business.
Keeping integrity preserved
Organizations have to remember that even when ML models have successfully been deployed at scale, there is still work to be done. The reason for this is that the data which underpins and drives these models is in constant flux, and the models need to respond appropriately. Once an effective ML model is implemented, there is an ongoing requirement to keep it fine-tuned and ensure it’s working efficiently. Processes such as these involve continual assessments of how the ML models are performing, how they are responding to changes and the impact this will have on the algorithms and business they serve in the future.
Narrowing the skills gap
When choosing the best team of people to work with and support ML models, companies should aim to create a team with capabilities, talents and experiences that cover an expansive range of skills sets. Organizations should include a mix of people in these teams; from product development and DevOps to data engineers and data scientists. That’s because a mixture of people will bring different knowledge levels and perspectives to every single project in motion, making sure the best results are delivered. The greater the diversity in the team, the more each member can learn from one another and develop together.
Approach models in production like living software
While it is important ML models are maintained, they must also be protected. It is paramount that businesses monitor who can access and make changes to the models as well as have visibility into model lineage. Implementing access restrictions ensures that exclusively those who should or need to amend ML models are able to do so. Following these steps will help upkeep accuracy and integrity – two fundamental elements to any effective ML model.
Upholding ethical standards
Last, but certainly not least, organizations must have ethical considerations at the top of their priority list when it comes to ML. To kick this off, businesses need to have consent from stakeholders and customers before incorporating their data into the ML model. By abiding to a meticulous set of ethical ML obligations early on, enterprises can avoid time being wasted and additional challenges arising from trying to retrofit ethical practices later down the line.
In adhering to and following these ten steps, organizations will put themselves on the path to reaping the benefits of ML, and taking their digital transformation to the next level. With spend in ML continuing to increase, there has never been a better time for businesses to invest in their ML models. As they do so, IT management teams and leaders must work collaboratively to ensure the right teams are established to; develop and grow ML models as the organization does, grant access only to those that need it and ensure that the integrity of the models are upheld. It can seem like a daunting task to implement ML, but armed with these ten steps, it doesn’t have to be. So long as companies are open to committing themselves to the process, the benefits of ML across the business are there to be reaped.
- We feature the best help desk software.
Contributer : Techradar - All the latest technology news https://ift.tt/2U7XUUw
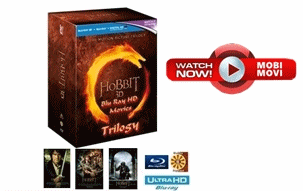

No comments:
Post a Comment